Latent Variable Models for Multifaceted Subspace Clustering
- 項目計劃:
- 優配研究金
- 項目年份:
- 2019/2020
- 項目負責人:
- 潘建文博士
- (數學與資訊科技學系)
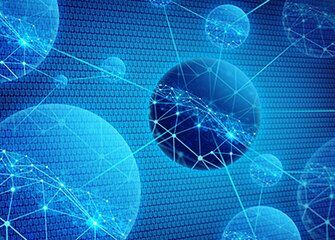
In this project, we aim to develop a solution for the problem of finding multiple clusterings of data points residing in different subspaces on a data set.
We have witnessed an explosion of data available from multiple sources and modalities. Although data sets today usually have a high dimension, it has been observed that their intrinsic dimension is often much smaller and that interesting patterns can be found in lower dimensional subspaces. Subspace clustering exploits the above observed property and finds groups of data points residing in different subspaces. It has found successful applications in various domains including image segmentation, motion segmentation, face clustering, and gene expression data clustering. Many of the recent subspace clustering methods, however, suffer from one major drawback: they attempt to find only a single partition of data and thus may not suffice to handle high-dimensional data that are often multifaceted. For example, while subspace clustering methods can accurately group face images based on subjects, it may ignore other meaningful groupings of face images such as those grouped facial expressions and/or by the existence of sunglasses. In this project, we aim to develop a solution for the problem of finding multiple clusterings of data points residing in different subspaces on a data set. We refer to such a problem as multifaceted subspace clustering. The solution will be based on probabilistic models due to their sound statistical basis. The proposed models will contain multiple discrete latent variables to represent multiple clusterings along different facets. To represent the local subspaces in each clustering, we will consider model structures based on conventional dimension reduction techniques. To represent the probabilistic relationships among the clusterings, we will consider two kinds of network structures with varying levels of complexity. We will develop inference algorithms as well as parameter and structure learning algorithms for those variants of models. The model variants will be evaluated and compared on various kinds of data sets where multiple meaningful clusterings are known to exist. The successful completion of this project will result in a solution for multifaceted subspace clustering, which currently receives insufficient research effort. A successful solution could find many meaningful applications in real world situations. It could attract more attention to this new research direction and could lead to future commercialization. Besides, the proposed models may produce better clustering performance over state-of-the-art subspace clustering methods and those producing multiple clusterings, respectively. Moreover, the proposed method can also automatically determine the numbers of clusterings and subspaces. It could be used for automatic categorization of vast amount data based on multiple aspects without any manual input.