Comparative Prosody Modelling across Languages
- 項目計劃:
- 優配研究金
- 項目年份:
- 2021/2022
- 項目負責人:
- 李烱樂博士
- (語言學及現代語言系)
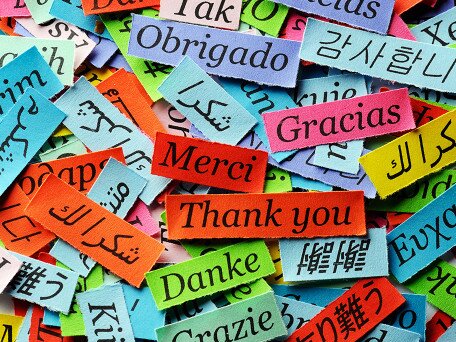
Two problems have remained unresolved in speech prosody research. The first one is that there are numerous rival theories that have coexisted for decades -- supporters for one do not necessarily understand the others well.
The second one is that in the absence of a universally accepted framework, field linguists working with a new language could propose prosodic analyses not otherwise satisfactory to fellow researchers, in part also due to field-related practical challenges compared with lab settings. Computational modelling can be a useful tool for addressing these problems.
This project seeks to promote computational modeling of fundamental frequency as a tool for (i) theory comparison and (ii) hypothesis testing and analysis *for field linguists*. Here we specifically target linguists without background in computer science or statistics.
In the first part of this project, we will compare two currently prevailing rival theories of speech prosody, namely the Autosegmental-Metrical Theory and the Parallel Encoding and Target Approximation Model, in terms of their respective synthesis accuracy. The two theories will be implemented using the PRAAT-based synthesisers AMtrainer, qTAtrainer and PENTAtrainer, and compared through identical sets of speech data of varying lengths, communicative functions (sentence types and focus), and languages (Cantonese and Fijian). Their relative performance in these conditions will illuminate the differences in theoretical assumptions and workings between the two models. The resultant synthetic stimuli will be verified by native listeners in perception experiments. The findings will lead to a better understanding of both AM and PENTA, enabling less experienced researchers to choose their preferred model based on their needs. As the first systematic study of Fijian prosody, this project will also provide precious first-hand data of this lesser-studied language.
The second part involves refining PENTAtrainer for enhanced synthesis accuracy. Although it has already achieved excellent accuracy (Pearson’s r > .9) in numerous previous studies, several known sources of remaining errors (i.e. pre-low raising and time-delay) await to be addressed. By incorporating these factors into PENTAtrainer, we can take its accuracy to an even higher level, opening up new research and practical possibilities.
The final part is a knowledge transfer project in which we seek to promote the use of PENTAtrainer among field linguists. We will create training materials (tutorial manual, website, and videos) targeting linguists in the field using materials yielded from the above. We hope this will pave the way for analysis-by-synthesis to become the next standard tool in language documentation.